

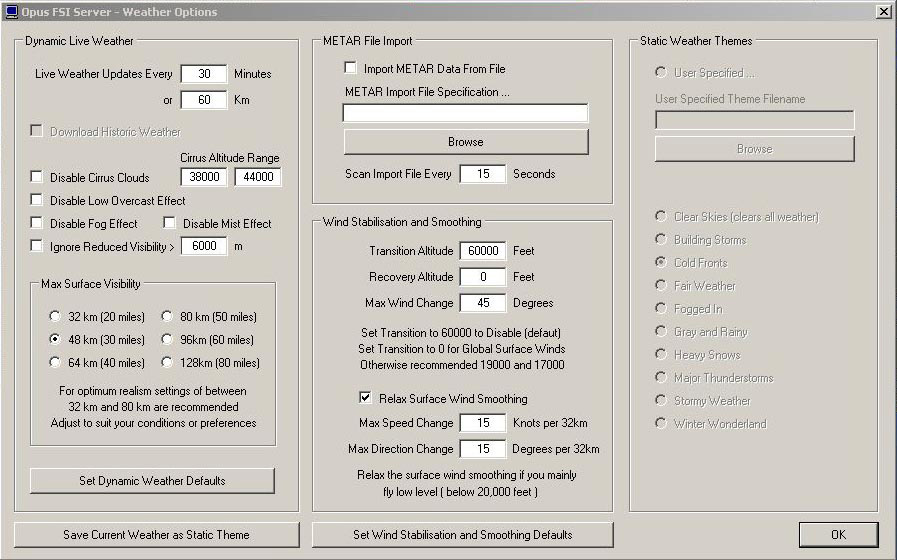

#Opus bitext and monolingual data full
We also conduct a comprehensive study on how each part in the pipeline works. Two approaches to make full use of the sourceside monolingual data in NMT are proposed using the self-learning algorithm to generate the synthetic large-scale parallel data for NMT training and the multi-task learning framework using two NMTs to predict the translation and the reordered source-side monolingUAL sentences simultaneously. Our approach achieves state-of-the-art results on WMT16, WMT17, WMT18 English$German translations and WMT19 German!French translations, which demonstrate the effectiveness of our method. Through extensive experiments on four lo w-resource language pairs. It can diversify the in-domain bitext data with ner level control. Finally, the model is fine-tuned on the genuine bitext and a clean version of a subset of the synthetic bitext without adding any noise. bitext without using any extra monolingual data explicitly. are connected with massively multilingual data sets and data-driven natural. Next, a model is trained on a noised version of the concatenated synthetic bitext where each source sequence is randomly corrupted. In this talk I will discuss the current state of OPUS-MT, our project on. This tech-nique was used to create the Paraphrase Database (Ganitkevitch et al. First, we generate synthetic bitext by translating monolingual data from the two domains into the other domain using the models pretrained on genuine bitext. monolingual tasks with bilingual pivoting (Ban-nard and Callison-Burch,2005), which assumes that two English phrases that translate to the same foreign phrase have similar meaning. Finally, we scale to hundreds of millions of monolingual sentences and achieve a new state of the art of 35 BLEU on the WMT’14 English-German test set. First, we generate synthetic bitext by translating monolingual data from the two domains into the other domain using the models pretrained on genuine bitext. We also compare how synthetic data compares to genuine bitext and study various domain effects.
#Opus bitext and monolingual data how to
In this work, we study how to use both the source-side and targetside monolingual data for NMT, and propose an effective strategy leveraging both of them. In this work, we study how to use both the source-side and targetside monolingual data for NMT, and propose an effective strategy leveraging both of them. Historically, parallel data were sourced from translations in multilingual public spaces like United Nations, European Parliament. While target-side monolingual data has been proven to be very useful to improve neural machine translation (briefly, NMT) through back translation, source-side monolingual data is not well investigated. Parallel data (bitext) The type of data that is needed to build a machine translation system is parallel data, which consists of a collection of sentences in a language together with their translations.
